Strategy
BOUNCE incorporates the most mature measures and outcomes in order to define the optimal prediction measures for understanding illness adaptation in breast cancer. Members of the consortium have considerable expertise in the assessment and analysis of parameters of well-being, survival, risk of metastasis, persistent pain and cognitive profiling. HUS, the coordinator of the BOUNCE project, has already a clinically validated and mature toolbox for predicting the patient-specific risk of sentinel node and non-sentinel node metastases in breast cancer. The specific models of HUS are also available as an application in the app store Predictive Tools for Breast Cancer. The ALGA-C and ALGA-BC (breast cancer psycho-emotional profiling) have been designed and clinically validated by the European Institute of Oncology in Milan and developed by FORTH as a predictive model for cognitive outcomes.
Within the clinical, psychosocial and computational framework described above, the multidisciplinary BOUNCE project is currently developing clinically acceptable models of patient successful adaptation to breast cancer, ultimately capable of providing clinical oncologists and health professionals with concrete recommendations regarding personalized patient management. An innovative feature of the BOUNCE project relies on an effective fusion strategy to boost the overall models’ accuracy. In addition, since the output of the models concerns multiple types of trajectories, weighted average schemes (e.g. linear combination, Bayesian combination, Bayesian Model Averaging (BMA), fuzzy rules, evidential reasoning technique) as well as machine learning-based methods will be applied. The fusion approach will also consider the different reliability factors of the models’ outcomes.
Initial modeling results from available data integrated over the first 6 months of the BOUNCE clinical study are very promising in supporting the transition toward a dynamically adaptable, personalized assessment and intervention planning tool suitable for routine clinical use, which takes into account all known potentially relevant parameters that may affect illness adaptation and resilience. Based on the extensive clinical experience and research track of BOUNCE partners in a number of multi-disciplinary fields, we have identified and aim to address a number of key obstacles to reaching this goal in the following domains:
- Definition of reliably measurable dimensionsof resilience.
- Objective assessment of the clinical validity of resilience attributesthrough conventional statistical approaches. This difficulty may be compounded by the dynamic, ever-changing nature of illness adaptation cognitions and behaviours and the complex interplay between contributing factors.
- Identification of key modifiable attributes of resilienceas a function of both individual characteristics (such as sociodemographic and cancer type) and ever-changing life circumstances that may affect the person’s dynamic adaptation process.
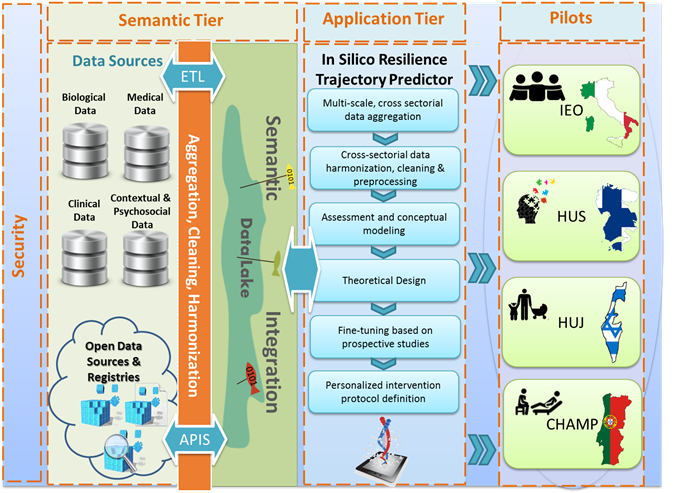